Potential of service-based business models for customer loyalty
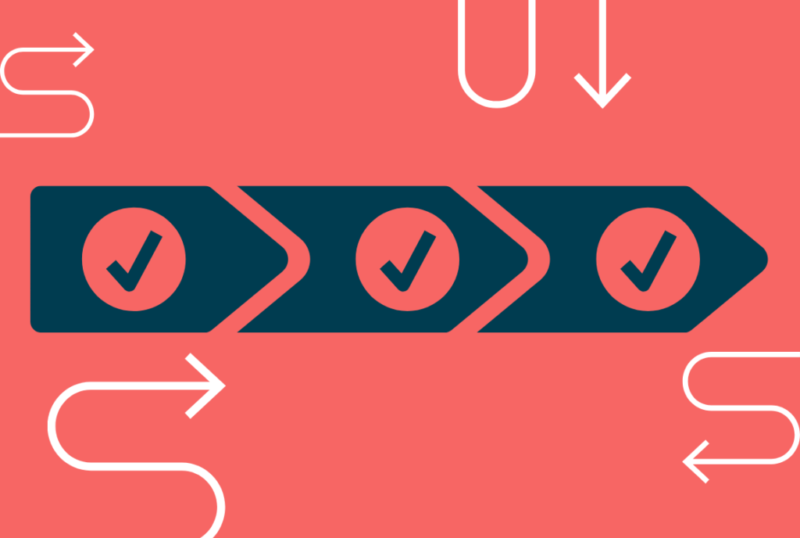
For B2B companies, customer acquisition can be quite challenging and requires a lot of operational and financial resources. Even for smaller deals, the sales process follows an iterative approach, starting with marketing engagement, followed by technical discussions, discovery and demo conversations, product-/ process iterations and ultimately proposals, which are hopefully followed by the approval from the relevant stakeholders. Moreover, the typical customer acquisition process is still mainly driven by offline activities such as the participation in fairs and trade shows, expert conferences and in-person meetings with (potential) customers. Additionally, the cost per online lead generation should not be underestimated and is up to eight times higher for manufacturing companies than it is for instance in retail.
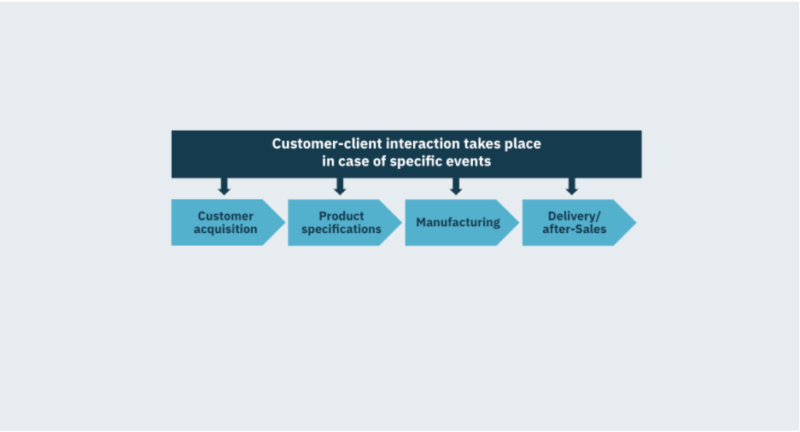
Consequently, customer acquisition binds substantial resources, whereas customer retention is seldomly given equal attention. After the transaction, customer interactions typically take place only in case of specific service or maintenance requests. Despite the well-known fact that customer retention is significantly more affordable and has a higher long term return on investment than a constant acquisition of new customers. Therefore, improving retention rates, which can be realized through an increase in customer satisfaction, can increase profits.
Actionable data thanks to value chain digitalization
The main motivation for manufacturing companies to digitalize the value chain is to improve process efficiency, to lower operational costs, to offer additional services to their customers and to lower the customer acquisition cost. A digitalized value chain and processes result in valuable data points that improve the continuous understanding of customer requirements and impact product development. As a result, manufacturers can increase machine sales, tap into new revenue channels and reach higher customer engagement levels, thereby gaining a competitive advantage.
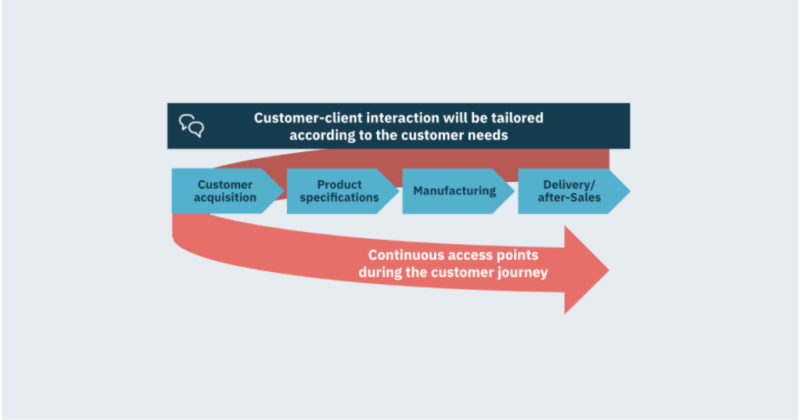
In order to kickoff the data-enabled digital process optimization, a deep data analysis that includes a number of streams is required. These include: correlation of complex control parameters, a feedback-based optimization cycle, consistency and high sensitivity of control and optimization and deep learning for continuous improvement of decision making. Particular care must be taken in this context to ensure that the potential risk of intransparency regarding optimization algorithms is addressed.
New technologies, powerful data frameworks and the combination of both open new ways for companies to establish service business models. These models improve customer satisfaction and boost retention rates. They also motivate new revenue channels based on new digital solutions and offer upselling potential for existing products. Even complex products, processes and systems can be simulated in their working environment, thereby decreasing development time, maintenance and operational cost. For example, a powerful combination using simulation-based digital twins with IoT data (from machines, manufacturing floors, etc.) allows for real-time assessments leading to innovative new service solutions and offerings. This combination, however, requires a multiple simulation model to predict the behavior of assets in real-world conditions.
Adoption of data-enriched and data-driven service business models
Once a company’s operations have been digitalized, the resulting data and insights lend themselves to monetization with data-enriched and data-driven service business models. Digitalization enables the real-time analysis of operational data and enables services to be adapted to customers’ needs. Initially, these data-enabled improvements were primarily used to optimize a company’s internal operations and the existing product portfolio. Subsequently, the move from one-time sales of a product to continuous service agreements led to the emergence of various Everything-as-a-Service (XaaS) models. These models enable manufacturers to provide and then charge for their products and services according to the availability (‘pay-per-hour’), productivity (‘pay-per-piece’) or functionality (‘pay-per- feature’).
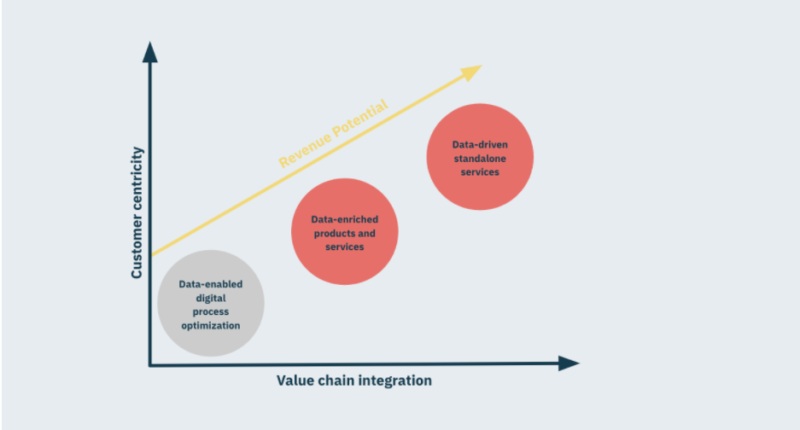
More and more traditional manufacturing companies are updating their business models and add data-enriched services to their product sales. At the same time, enhancing the ability to measure the outcomes of the services they deliver. This trend is facilitated by sensors and connectivity becoming ubiquitous in a growing number of environments. Sensor data can be used to identify bottlenecks in the supply chain, assess the quality of materials and offer new services like predictive maintenance and auto replenishment. The move from product sales towards subscription models helps companies to achieve deeper value chain integration, thereby positioning their businesses closer to their customers. From a customer perspective, business models that sell results appeal far more than those that just sell products. Manufacturing companies can thereby actively address the average customer retention rate of around 67 percent, which makes manufacturing an industry with one of the lowest rates.
A prime example of the value of data-driven service business models is Schindler, Swiss manufacturer of elevators, escalators and moving walkways. The company equips its elevators with IoT sensors that measure parameters such as load weighing, number of trips, number of door cycles, waiting times, acceleration, jerk, bumps, vibration and others. This proved to be advantageous to the company and its customers by improving the preventive maintenance schedule and offering additional services.
Zeiss, together with Microsoft, go even further by working on a data-driven stand alone service: As part of the company’s transformation into a digital services provider, the company will standardize its equipment and processes on Azure, Microsoft’s cloud computing platform. The company will be able to provide its customers one digital platform to manage all digital Zeiss products.
Servitization enables companies to continue successfully into the future
To summarize, data-enriched and data-driven service models require a digitally-enabled process optimization to be effective and purposeful. The deeper value chain integration calls for fundamentally different skill sets than the traditional domain knowledge. As a consequence, the complexity of building impactful service business models based on data should not be underestimated. From an organizational perspective, the process involves the implementation of an iterative and agile software development environment and culture with a recruiting strategy designed to find the right talents for data-driven service development. From a strategy perspective, the required data strategy framework needs to be considered in the company’s investment and overall strategy. To maximize the potential posed by data-driven service business models, companies need to understand it as a long term engagement, which is underpinned by a data-driven product strategy and a data-driven or data-supported sales framework.
Recent findings reported by McKinsey show that COVID-19 further increases the servitization of manufacturers’ business models and delivery. So far, the pandemic caused 96 percent of B2B organizations to shift their operating model to emphasize digitally enabled self-serve, remote and contactless operations. This includes virtual delivery models as well as the increased use of digital self-serve and remote solutions.