The human side of AI: success factors for transformation
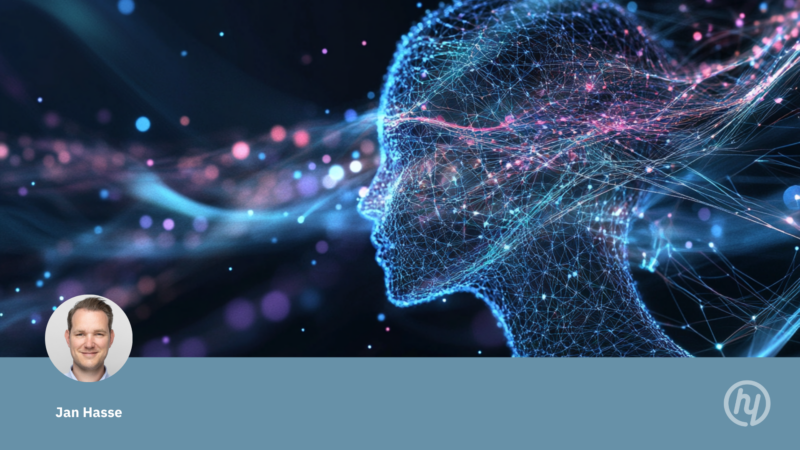
Successful AI adoption requires more than technological expertise. This article shows why strategic focus, targeted re- and upskilling as well as effective governance are essential. It also explains which organizational models can help leaders and those responsible for HR, Learning & Development and People & Organization to successfully drive their AI transformation journey.
1. Shaping the future: AI as a driver of innovation and change
Innovation is accelerating. In a world where technological cycles are rapidly shortening, global innovation hubs, BigTechs and visionary minds from the start-up scene are setting disruptive impulses. We are on the threshold of an era in which data-driven and AI-fueled processes and products will become central priorities for corporates and SMEs – driven by exponential technologies that create new business models and new growth opportunities.
But where does Germany stand? Corporates and SMEs often struggle to apply AI and to successfully implement transformations. This knowledge is based on more than a decade of my own experience, projects at Axel Springer hy to develop future-oriented organizational structures, our joint study with Duke University, and intensive discussions with executives and HR experts.
One finding stands out: the problem rarely lies with the technology alone, but rather with the ability to integrate and successfully implement AI strategically. Above all, there is a lack of talent, knowledge and skills to fully exploit the potential of AI and make it usable in the long term.
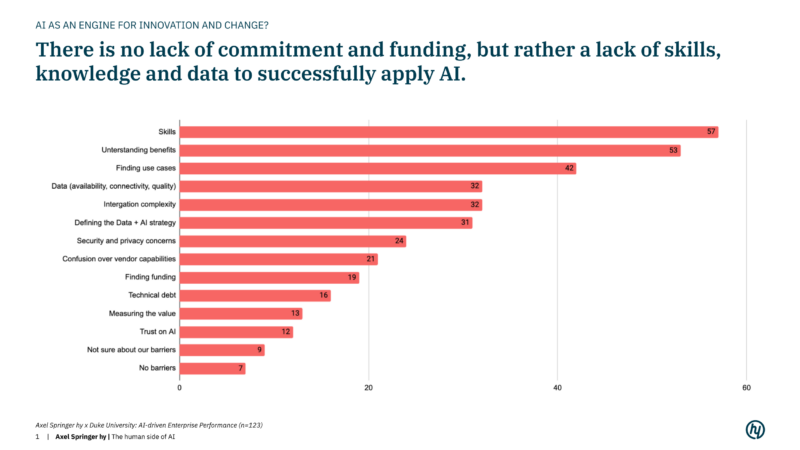
Other studies also confirm the need to catch up. In 2026, an additional 780,000 skilled workers with digital, technological and transformative “future skills” will be needed in Germany (Stifterverband and McKinsey, 2021). Above all, the demand for data analysts and AI experts is increasing enormously (Stepstone, 2024). Completely new roles also require the adaptation of the organizational structure (Haufe, 2024). Without this organizational change, progress, innovative strength and the recruitment of new talent will be significantly slowed down. The studies make it clear that we need to broaden our perspective from pure technology to comprehensive transformation, with a focus on three central aspects:
Organizational Change: Creating the structure for change
AI requires new roles, interlinked end-to-end processes and the next generation of leadership approaches. Companies need to reorganize themselves to make AI a core part of their strategy.
Cultural change: Empowering and inspiring people
An open dialog about opportunities and fears is essential. Employees must not only be activated, but also inspired and empowered, so that they perceive AI as a tool to support their work and use it effectively.
Human first: Creating acceptance through benefits
The question is not whether AI replaces people’s work, but how it optimizes it. Trust and acceptance only comes when AI is seen not as a threat, but as a support and an enabler.
How can we design efficient and effective AI governance that acts as a unifying element between technology, strategy, structure and people, and that focuses on culture? It should not only optimize existing business processes, but also promote strategic growth, prepare employees and managers for new roles, train them in a targeted manner and enable comprehensive, sustainable change.
2. What Digital Transformation has taught us
The challenges of AI transformation lie less in the technology itself than in the strategic, organizational and cultural prerequisites. This insight is not new: digital transformation has already shown that success is only possible with a holistic approach. So how can we learn from past experiences and apply these lessons to AI transformation?
A strategy with vision: providing direction instead of improvising
Successful digital transformations were characterized by clearly formulated and measurable strategies that aligned business objectives, available resources, and timelines. These strategies were not only technologically driven, but also organizationally focused. The parallel to AI transformation is clear: an integrated data and AI strategy is the compass that guides organizations through complex change processes.
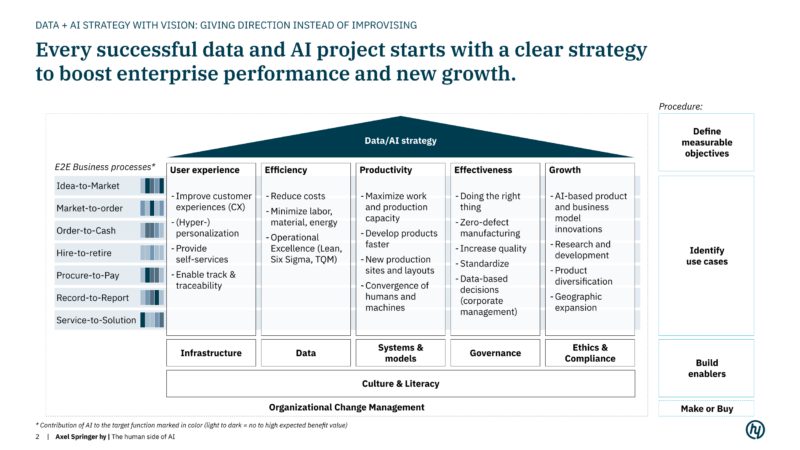
Companies significantly increase their chances of success with a precise strategic orientation. This strategy must combine short-, medium- and long-term perspectives, identify and prioritize concrete potential, and create the necessary conditions for it.
Leadership as a driver of change
A key success factor in the digital transformation was the commitment of top management. Leaders who acted boldly, supported digital technologies and were willing to challenge old paradigms created the baseline for success. The same applies to the AI transformation: leadership means not only promoting technological innovation, but also actively guiding it and acting as a role model for cultural change. A willingness to change at the management level is a prerequisite for success. Without this commitment, any strategy remains a paper tiger.
Human first: Change needs culture
Employees not only want to be informed, but also actively involved in change. The workplace of the future will be characterized by agile, cross-functional teams organized based on skills rather than rigid hierarchies or roles. For AI transformation, this means that cultural change is not a sideshow, but a core part of AI transformation journeys.
AI teams must work in a diverse and collaborative way to develop and scale relevant use cases. Data-driven knowledge management and measurable KPIs are indispensable tools for making successes visible. As with the digital transformation, the acceptance of technology depends on how well it supports and develops employees in their work.
Skills as the currency of the future
The importance of digital literacy is increasing because the introduction of new key technologies can only succeed if the workforce is prepared to use them. For the AI transformation, this means promoting data and AI literacy and, in connection with this, a better understanding of data-driven processes and the ability to use AI productivity tools effectively. Employees must be empowered not only to recognize the potential of AI, but also to actively adopt it. Continuous re- and upskilling based on individual skills and requirements from updated role profiles and job descriptions will be a top priority for HR in 2025.
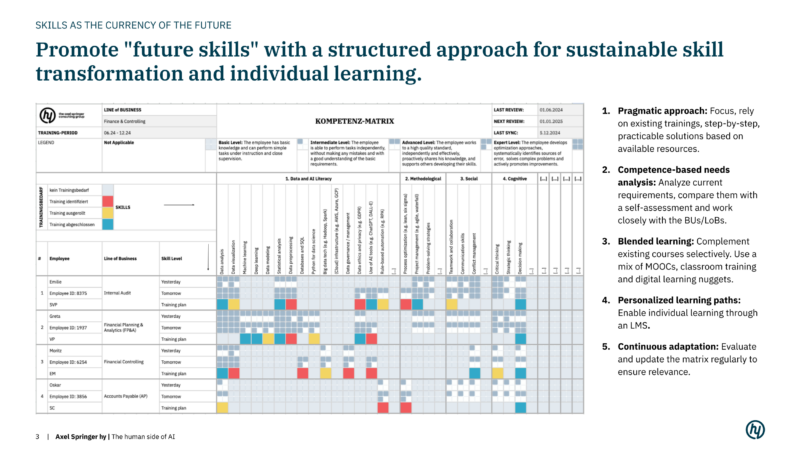
Flexibility as a basic principle
The ability to respond agilely to change was a key factor in the digital transformation. Companies that retained rigid structures were overtaken by more dynamic competitors. This applies even more to the AI transformation: it demands flexible organizational structures that can be continuously adapted. An adaptive mindset is crucial to respond to the rapid development of technologies. Companies that act with agility have a clear advantage.
The bottom line: governance as a critical success factor
One lesson that literally stands out from these five points is the central importance of governance. Digital transformation has shown that isolated roles such as a Chief Digital Officer (CDO) are not enough to guarantee sustainable success. Similarly, a Chief AI Officer (CAIO) will only be one building block of a successful AI transformation.
What is needed is an integrated AI governance that not only combines technological, but also strategic, organizational and cultural factors. It must manage change at all levels – from strategic planning to operational implementation – while ensuring that ethical, legal and economic requirements are met.
3. No “one-size-fits-all”: organizational models for AI transformation
Choosing the right organizational model for AI transformations is a highly individual decision. It depends not only on the organization’s AI maturity but also on its strategic goals, existing structures and the chosen implementation strategy.
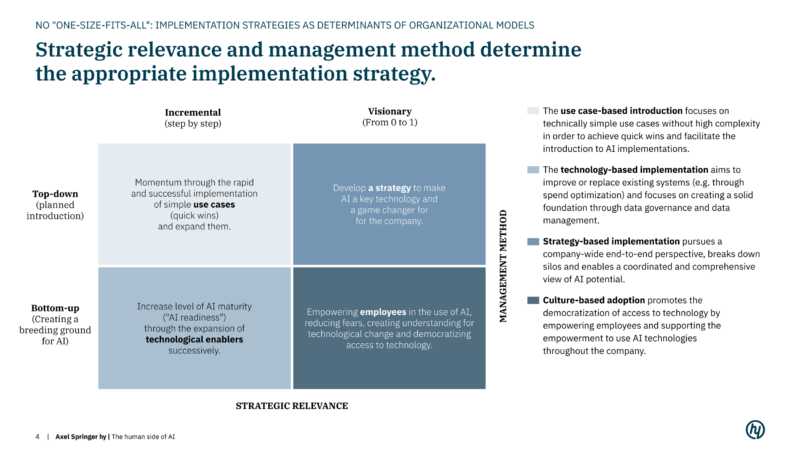
There is no perfect model that fits all organizations equally well. Instead, our project experience shows that a combination of different approaches is often the key to success.
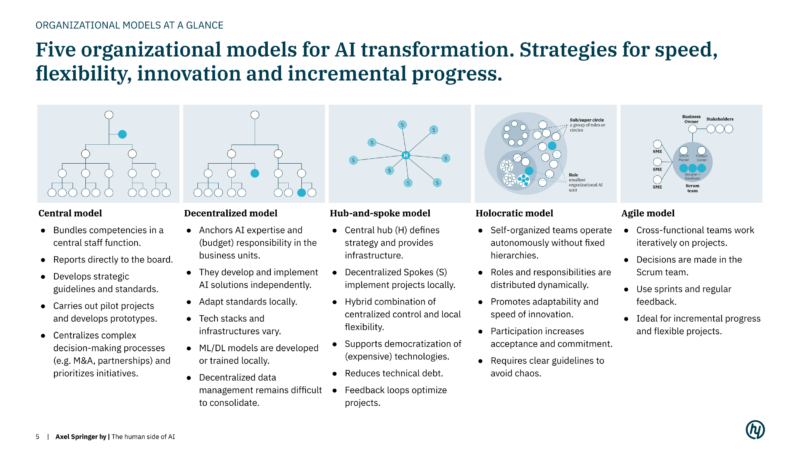
Central model
A central staff function, working group or center of excellence (CoE) is well suited to a strategy-based AI implementation because it enables the development of company-wide guidelines and standards. With a centralized budget and close proximity to senior management, it is particularly suited to quickly implementing disruptive ideas. This model is suitable for starting the AI transformation in companies of any size that are focusing on strategic growth areas.
Decentralized model
The decentralized model is most likely to support a technology-based AI implementation because it enables teams in business units to develop individual solutions and create the specific (technical) conditions for them, although “shadow IT” and redundancies in the infrastructure must be avoided. It is particularly suitable for companies with heterogeneous structures and a focus on increasing efficiency, productivity and reducing costs.
Hybrid hub-and-spoke model
This model combines a strategy-based and technology-based strategy, as the hub takes over central control and resource management, while the spokes adapt and implement local projects. It is particularly suitable for companies with established AI initiatives that want to scale. Ideal for corporations with multiple business units that want to combine centralized support for infrastructure and disruptive investments with local flexibility for optimization measures.
Holacratic model
The holacratic model is an excellent fit for culture-based implementation because it strengthens acceptance through maximum participation and personal responsibility. It is particularly suitable for startups, scaleups or companies with a strong focus on R&D projects. It is used in dynamic, innovation-driven organizations that prioritize cultural change.
Agile model
The agile model is ideal for use case-based introduction because it enables rapid results through iterative development and regular feedback. Combined with lean approaches and design thinking, it is particularly suitable for specified development projects in companies of all sizes that rely on incremental progress in short sprints and flexibility.
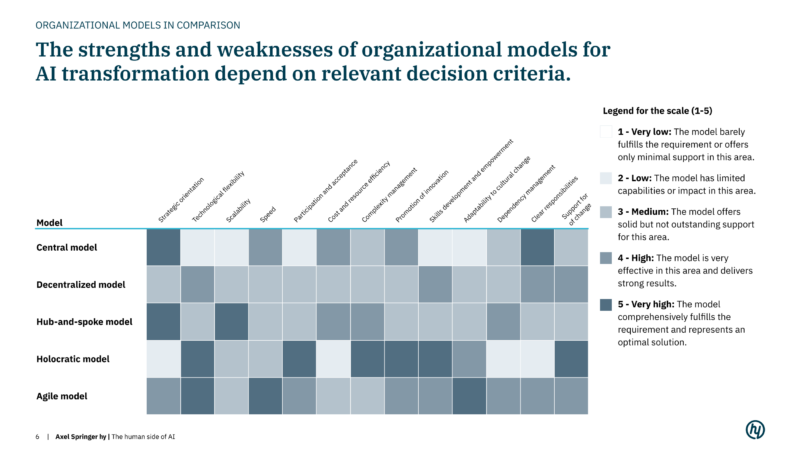
The models presented provide a simplified orientation for designing the AI transformation. Each model brings specific strengths that can be ideally deployed depending on the context and requirements of a company. It is crucial that organizations carefully assess their individual needs, resources, and cultural circumstances. Flexibility and a willingness to adapt structures to dynamic developments are indispensable.
In practice, a successful AI transformation often requires hybrid approaches. For example, a company could start with a central working group to define strategic guidelines, implement initial lighthouse projects and increase AI maturity through training and high-performance infrastructure. As experience grows, decentralized teams take over scaling, while the nucleus provides governance and support. Agile teams could implement prioritized projects at high speed, while a hub-and-spoke model ensures centralized control of infrastructure and local adaptation. This creates a flexible, high-performance system for the sustainable success of the AI transformation.
4. Shape the change: HR as a driver of AI transformation
Choosing the right governance structure requires an analysis of the individual company’s goals. Strategic focus is crucial: a clear AI strategy helps set priorities and allocate resources efficiently. A few, but impactful use cases are the key to rapid value creation and long-term innovation. There is no standard solution – flexibility and context sensitivity are essential.
However, the introduction of AI is not a purely technological challenge, but a comprehensive transformation that affects all areas of the company and therefore assigns an important role to Human Resources & Organization, Learning & Development and Executive Development, which will face three important tasks in 2025:
Strategy development and activation:
Develop a clear, targeted AI strategy together with company management and the strategy department. Support this process by designing and conducting appropriate workshop formats. These should activate managers and employees, transfer knowledge, make AI tangible and provide a suitable format for identifying use cases.
Competency development and cultural promotion:
Start by analyzing current and future competency requirements. Put existing job descriptions to the test now. Every job will be fundamentally changed by AI and new role profiles will emerge. Develop targeted training programs based on a skills matrix that enable individual learning paths and improve data and AI literacy in your organization. The goal is to empower employees, reduce fear, and foster an innovation-driven culture in which AI is perceived as a supportive tool.
Establish structures and alignment:
Choose an organizational model that fits your company’s strategic goals and AI maturity level. Create the necessary structures to effectively implement your strategy. Make sure you work closely with technical functions such as IT, ISMS, the CISO and the Data Protection Officer to take technological and regulatory requirements into account from scratch.
A swift implementation will be key to retaining your employees, attracting new talent and successfully creating the future. AI transformation starts small but requires a clear vision – driven by courageous and visionary leaders and a dedicated HR team. Are you looking for an AI skills matrix, need support in executing your AI transformation journey or would like to challenge individual tasks or steps with us?
Get in touch!