AI-based pricing – from vision to concrete implementation
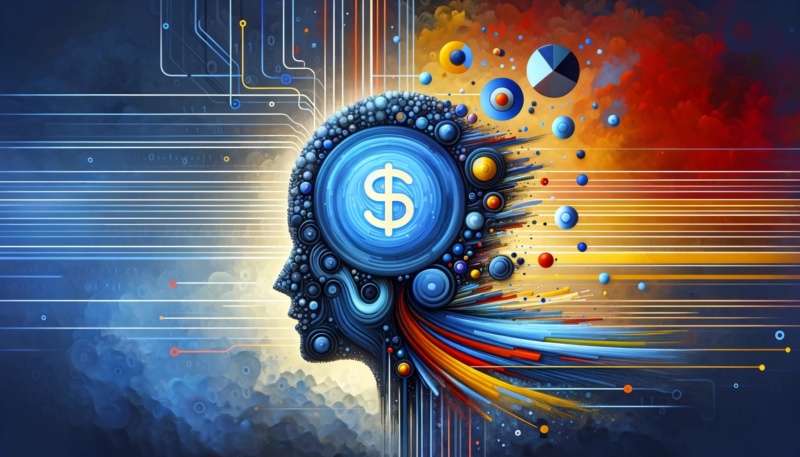
“Think big, start small, scale fast” – A guide
Artificial intelligence (AI) is on everyone’s lips and has become a hype topic in many companies since the introduction of ChatGPT at the end of November 2022. The media attention for AI is enormous and the potential use cases are diverse. As a result, the discussion of AI-based applications in pricing has also become considerably more important in recent months. A look at current developments quickly shows that AI is a highly relevant topic in pricing: The market for price optimization software is experiencing dynamic growth and is expected to reach $2.71 billion by 2028, supported by increasing interest in Data Science for AI-driven pricing. At the same time, there is a steady increase in scientific publications on this topic. Despite the current low use of generative AI at 3% among German companies, a significant increase of 200 % is planned by 2024 (source, source, source, source).
Despite the growing interest in AI pricing, there is often a lack of clear initiatives that actually increase profitability. Initial euphoria is quickly followed by disillusionment. It’s not easy to get to grips with the topic: many research efforts lead nowhere or to providers of half-finished software solutions, some of which promise significant double-digit increases in earnings in a short period of time. There are rarely any clear recommendations for action. Moreover, the major challenges concerning the topic are often glossed over. Indeed, approximately 80% of all AI models ultimately are not deployed (source).
In this article, we would like to take a systematic approach to the topic of AI in pricing and first look at the opportunities and challenges before discussing the major use cases of dynamic pricing and personalized pricing. We will then outline a process that helps to break down the big visions into concrete work packages. Finally, we will give ten practical tips on what to look out for when starting to deal with AI pricing in order to increase the chances of successful implementation.
The opportunities are huge, but so are the challenges
AI in pricing refers to the application of artificial intelligence in the field of pricing. Through extensive and automated data analysis, it enables highly precise and dynamic price adjustments and adapts efficiently to markets. This advanced technology provides companies with the opportunity to significantly increase their revenues and profit margins (source) by adjusting prices in real-time and accurately to changing market conditions.
Furthermore, AI enables a more efficient utilization and allocation of resources, leading to optimized usage. The precise adjustment of prices based on real-time data results in better control of supply and demand, leading to more efficient resource utilization and a reduction in overcapacity.
Increasing the Customer Lifetime Value is a crucial advantage of AI-based pricing. By analyzing individual behavioral patterns and preferences, the technology allows for personalized offers tailored to the needs of each customer. This not only enhances customer satisfaction but also strengthens the long-term relationship between companies and customers.
Another value-adding aspect is that AI enables real-time data-driven decision-making. Companies can make informed decisions based on precise market analysis and forecasts, increasing agility and responsiveness to market changes, contributing to strengthening their competitive position (source).
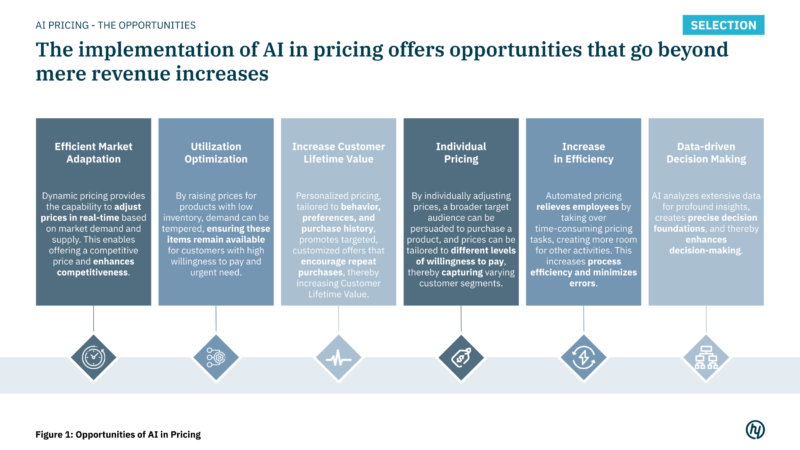
Alongside the diverse opportunities that AI in pricing offers, there are also some challenges to overcome.
Firstly, it is essential to note that the results achieved with AI are only as good as the underlying data. Therefore, companies must ensure that their data is of high quality to enable informed pricing decisions. Inadequate data quality or incomplete data (e.g., data on customer usage behavior, competitive prices) can lead to faulty price proposals and disadvantages for the company.
The integration of AI-based pricing into an existing organization requires linking various business units. This involves not only technical integration through IT interfaces but also the development of skills and the promotion of collaboration between employees from different departments, such as the pricing team, data science, product management, marketing, and sales.
Additionally, companies need to consider not only the costs of the new technology (whether software solutions or in-house solutions) but also the requisite expertise. This expertise can be acquired through either training initiatives or by bringing in qualified professionals.
Lack of transparency and communication regarding the reasons, timing, and nature of price changes, as well as significantly different individual prices, can be perceived as unfair and affect customer satisfaction. For instance, following the announcement of the implementation of dynamic pricing, a fast-food chain encountered significant customer backlash, ultimately prompting a widespread boycott (source). Consequently, the chain opted to abandon this initiative (source).
To prevent potential margin erosion due to aggressive pricing strategies and rapidly changing markets, it is also recommended to monitor market trends and regularly update models to adapt to changing market conditions.
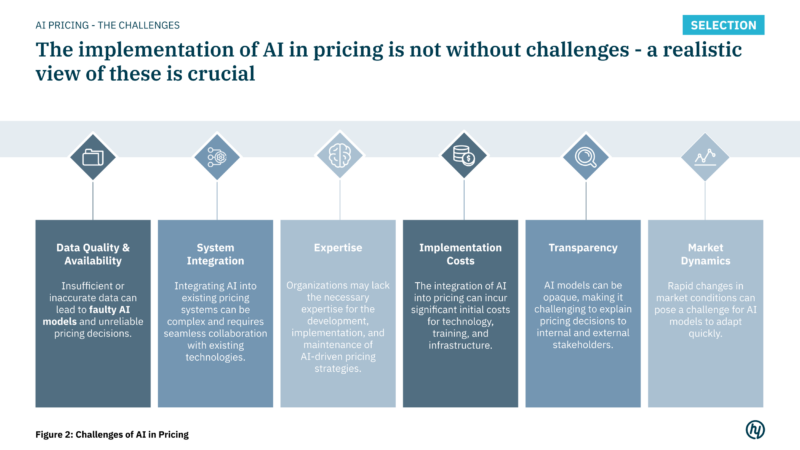
Despite the challenges, the strategic utilization of AI in pricing presents opportunities that far outweigh these obstacles. However, it is crucial for companies to act thoughtfully to fully benefit from AI in pricing. Therefore, it is key to define use cases for a company and to systematically address them.
Dynamic Pricing and Personalized Pricing are the major AI pricing strategies
From conversations with our customers, we know that use cases for the use of AI in pricing are quickly identified. No wonder: examples from the airline industry (source), where (it seems like) everyone pays a different price, or from Amazon (source), which adjusts prices 2.5 million times a day, are widely recognized by the general public. This quickly leads to ideas for implementing AI in one’s own company. Dynamic Pricing and Personalized Pricing are by far the most frequently mentioned keywords in the context of AI in pricing . Both strategies utilize data analysis and machine learning to adjust price decisions in real-time but differ in their application and objectives.
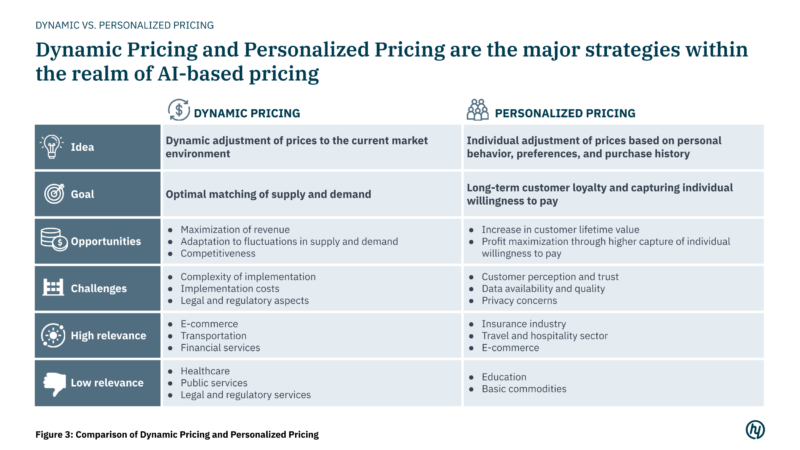
Dynamic Pricing refers to the real-time adjustment of prices based on market conditions such as supply, demand, and competitive prices. The goal is to dynamically optimize prices for products or services, inventory, or capacity to maximize demand or revenue. Dynamic Pricing is particularly useful in a volatile market environment with high demand fluctuations and intense competition, combined with short product life cycles.
Personalized Pricing involves the adjustment of prices for individual customers or customer segments based on their behavior, preferences, and purchase history. The goal is to personalize pricing to maximize Customer Lifetime Value, strengthen customer loyalty, and ultimately increase revenue and profit. Personalized Pricing is especially effective in environments where companies have detailed customer data and customers have high expectations for customized offers. It is suitable for markets where willingness to pay varies significantly, and customers receive individualized offers.
In summary, Dynamic Pricing focuses on external market conditions, while Personalized Pricing focuses on individual customer behavior. In practice, a combination of Dynamic and Personalized Pricing often occurs as the example of Uber shows. Their prices react extremely dynamically to minimal changes in demand and adapt for example to specific routes. Thus, the average price on routes with customers with high willingness-to-pay may be higher than elsewhere. Additionally, behaviors such as the willingness to accept higher prices of people with low battery levels on their phones are analyzed (source).
From Vision to Concrete Use Case
Behind the buzzwords Dynamic and Personalized Pricing, there is a broad range of application possibilities. Personalized Pricing, for example, can mean the simple introduction of a student discount at one end of the spectrum, while at the other end, it includes sophisticated AI-based pricing models that theoretically allow for a different price for every individual customer. Therefore, it is not enough to simply announce, “We are implementing Personalized Pricing now.” It requires a thorough analysis and strategic implementation to successfully overcome the various challenges. To get closer to this vision, a five-step process can help. This process assists in progressing from the current situation to the practical implementation of real use cases.
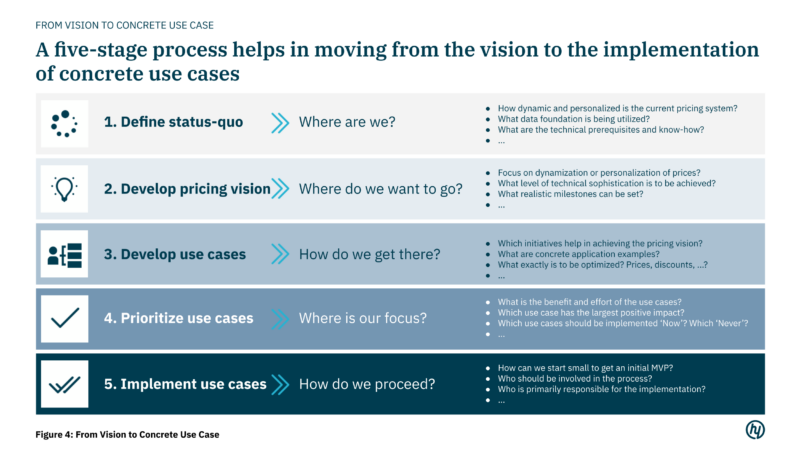
1. Define status-quo
A starting point for the use of AI in pricing lies in a comprehensive analysis of the current situation, encompassing existing pricing, available data, internal know-how, and technical requirements. The focus of this analysis is to check to what extent the current pricing system is already dynamic and personalized. It should be considered whether the existing knowledge and technical status in the company are sufficient and provide a solid foundation on which AI-based pricing can build. Additionally, considerations should be made regarding the time frame for project implementation and the planned investment volume.
2. Develop a pricing vision
The next step is to define a vision. Here, sensible prioritization is necessary: should the focus be on Dynamic or Personalized Pricing? The idea of introducing a comprehensive AI model in pricing that is entirely dynamic and personalized may be tempting. However, it is crucial to take one step at a time. Experience shows that the majority of value is created when taking small steps. While the vision of a perfect pricing system, as used by pioneers in this field, is appealing, it is advisable to formulate realistic interim goals alongside a grand vision.
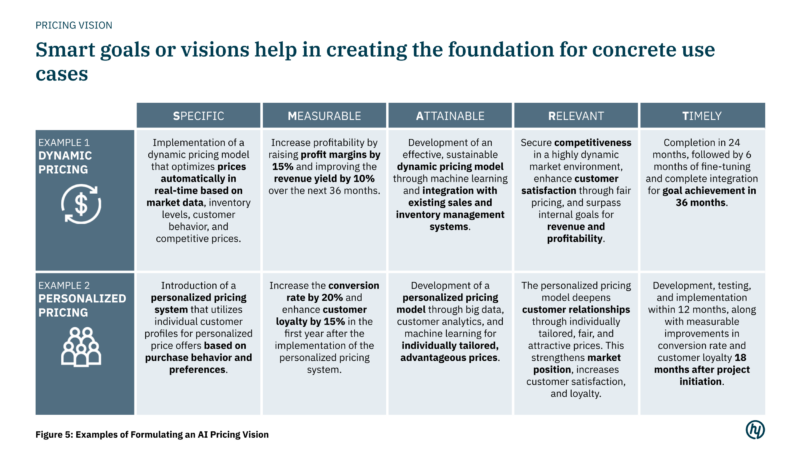
3. Develop use cases
Concrete use cases serve as meaningful interim goals, considering both the current situation and the vision. Let’s assume the goal is to implement Personalized Pricing. Although this vision may seem simple and generally acceptable at first glance, significant questions arise during specification:
- Which customer groups should the personalization target—new customers, existing customers, or both?
- Should prices or discounts be personalized?
- In which distribution channels should Personalized Pricing be applied?
These questions emphasize the need to define the use case as precisely as possible to avoid misunderstandings and have a clear direction for implementation. For example, the question whether to personalize for specific customer groups needs to be answered. In practice, there is a fundamental difference between whether personalization is aimed at new or existing customers. For existing customers, there may already be numerous data points such as location, purchasing behavior, price sensitivity, payment information, etc. For new customers, the data situation is usually much more limited.
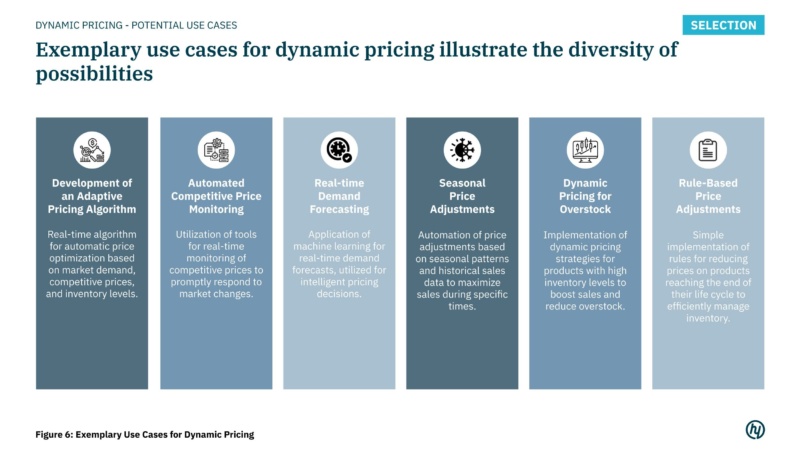
4. Prioritize use cases
Once use cases are defined, the next step is to prioritize them. The prioritization of use cases should be based on an impact-effort analysis. This involves not just financial evaluation criteria such as the Return on Investment, but a holistic view and assessment of the use cases. Figure 7 shows a selection of elements that can be included in the assessment.
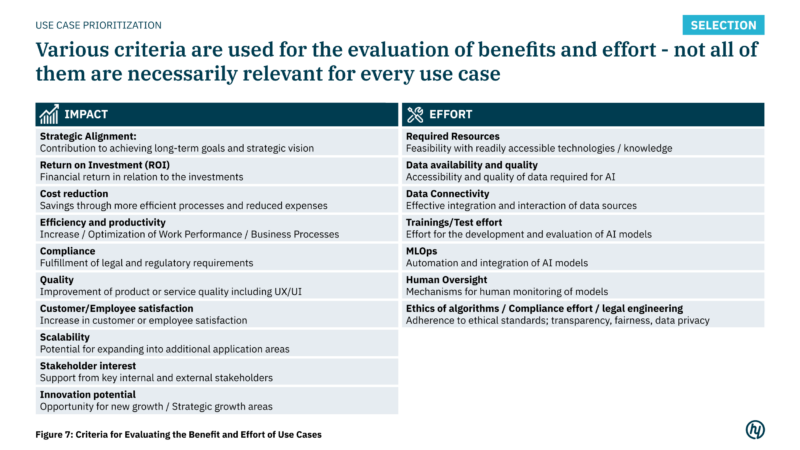
The impact and effort assessment is then plotted for each use case on a matrix. This provides a clear picture for prioritization (see Figure 8). Ideally, prioritize use cases with a significant positive impact and high technical feasibility. Practical experience shows that many optimizations occur in the ‘Now’ area (Low effort / High impact), while strategic growth topics are more likely in the ‘Next’ area (High effort / High impact).
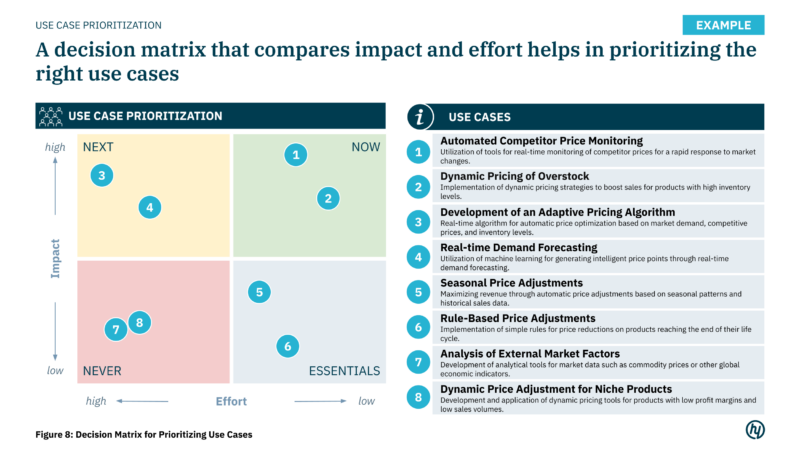
5. Implement use cases
After evaluating and prioritizing relevant use cases, the implementation begins. The significance of this phase becomes apparent when considering that that only about 20% of AI-based models are actually used in the end (source). From the beginning, all relevant areas of the company should be involved in the process to work towards a common goal. This includes not only the responsible product and data science teams but explicitly also sales, marketing, customer service, and finance. The responsible data product manager coordinates collaboration and ensures that all areas of the organization are prepared for the changes, facilitating smooth implementation (source).
Depending on the use case, the question often arises during implementation of whether a tool should be externally sourced (‘Buy’) or internally built (‘Build’). By now, there are already various standard solutions for simple pricing AI applications. Therefore, the decision for ‘Buy’ is often made for highly standardized use cases, such as the previously prioritized tool for automated competitive price monitoring (see Figure 9). However, the decision always depends on the specific company. If there is no time pressure, high strategic importance, special requirements, and the resources are also available, the decision can also be in favor of ‘Build’.
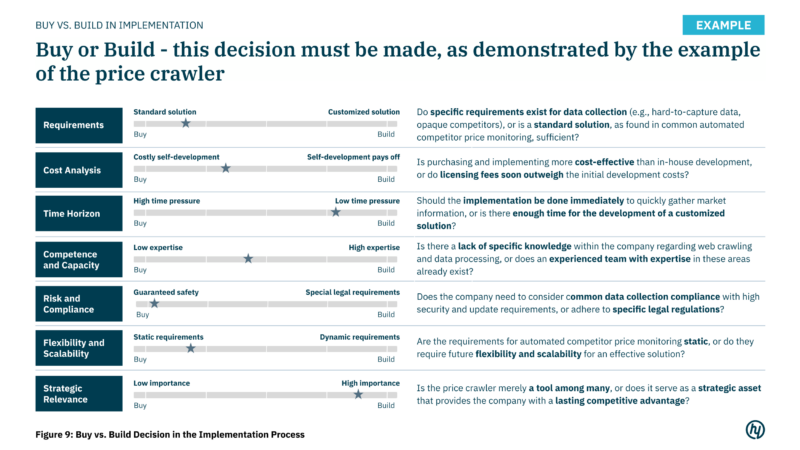
In general, it will be unavoidable that errors occur during the integration process. The key is to learn from these mistakes and make the necessary corrections. An agile approach allows continuous improvements. The previously outlined five-step process is considered iterative and must be repeated over time.
A core mistake is not getting started
From our perspective, it is crucial for any company dealing with pricing to understand the potentials of this new technology and to appropriately align it with their own interests. To remain competitive, the principle of ‘just do it,’ whether in dealing with the subject or implementing specific use cases, applies.
To facilitate the start, we have compiled ten practical recommendations to ensure that the focus is initially on the right topics see Figure 10.
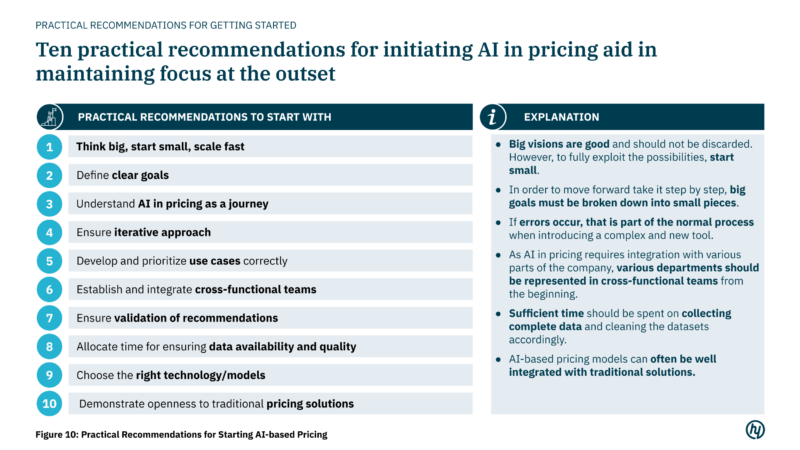
The principle of ‘Think big, start small, scale fast’ forms the foundation for successful implementation. It encourages companies to think big but start with small, manageable use cases that allow for quick scalability.
Defining clear goals and vision is crucial to avoid getting lost in the discussion of AI in pricing and to ensure alignment with the company’s business strategy. It is important to understand AI in pricing as a continuous, iterative process that requires adjustments and learning over time. This process involves prioritizing the right use cases, which should be selected based on an impact and effort assessment.
Finally, it is advisable for companies to maintain openness to traditional pricing solutions. Although AI in pricing offers numerous advantages, an immediate implementation is not always the optimal solution. In many cases, significant added value can already be achieved with simple approaches, such as segmenting prices for different target groups. Therefore, openness to non-AI-based pricing solutions should be sustained. It is expected that standard solutions in the market will continue to evolve in the coming years. Nevertheless, it is clear: companies that are already wisely investing in AI pricing today are gaining a strategic competitive advantage. Therefore, it is all the more important to engage with AI-based pricing and develop a well-founded strategy for dealing with it.
If you want to learn more about whether AI-based pricing is right for your company and how to use it optimally, feel free to contact us. We are happy to assist you.
*We would like to thank Olivia von Rudzinski for her contributions to this article.